How to show the equivalence between the regularized regression and their constraint formulas using KKTThe proof of equivalent formulas of ridge regressionRidge regression formulation as constrained versus penalized: How are they equivalent?Equivalence between Elastic Net formulationsCalculating $R^2$ for Elastic NetEquivalence between Elastic Net formulationsWhy is “relaxed lasso” different from standard lasso?Bridge penalty vs. Elastic Net regularizationLogistic regression coefficients are wildlyHow to explain differences in formulas of ridge regression, lasso, and elastic netIntuition Behind the Elastic Net PenaltyRegularized Logistic Regression: Lasso vs. Ridge vs. Elastic NetCan you predict the residuals from a regularized regression using the same data?Elastic Net and collinearity
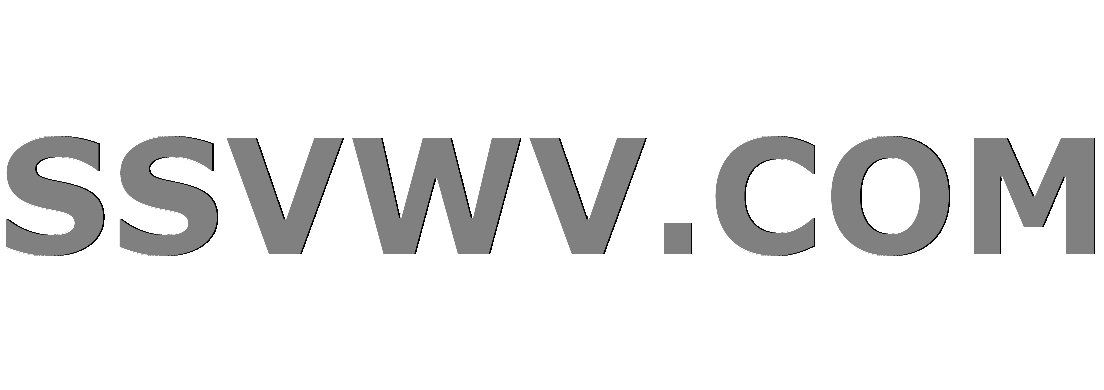
Multi tool use
Why are electrically insulating heatsinks so rare? Is it just cost?
Did Shadowfax go to Valinor?
How much of data wrangling is a data scientist's job?
UK: Is there precedent for the governments e-petition site changing the direction of a government decision?
Theorems that impeded progress
How can I make my BBEG immortal short of making them a Lich or Vampire?
Will google still index a page if I use a $_SESSION variable?
What mechanic is there to disable a threat instead of killing it?
What is the word for reserving something for yourself before others do?
Why is the 'in' operator throwing an error with a string literal instead of logging false?
I'm flying to France today and my passport expires in less than 2 months
How to take photos in burst mode, without vibration?
What does it mean to describe someone as a butt steak?
Can I use a neutral wire from another outlet to repair a broken neutral?
What is the PIE reconstruction for word-initial alpha with rough breathing?
Stopping power of mountain vs road bike
Arrow those variables!
In Romance of the Three Kingdoms why do people still use bamboo sticks when papers are already invented?
How to draw the figure with four pentagons?
If human space travel is limited by the G force vulnerability, is there a way to counter G forces?
Why can't we play rap on piano?
In a Spin are Both Wings Stalled?
I Accidentally Deleted a Stock Terminal Theme
What is going on with Captain Marvel's blood colour?
How to show the equivalence between the regularized regression and their constraint formulas using KKT
The proof of equivalent formulas of ridge regressionRidge regression formulation as constrained versus penalized: How are they equivalent?Equivalence between Elastic Net formulationsCalculating $R^2$ for Elastic NetEquivalence between Elastic Net formulationsWhy is “relaxed lasso” different from standard lasso?Bridge penalty vs. Elastic Net regularizationLogistic regression coefficients are wildlyHow to explain differences in formulas of ridge regression, lasso, and elastic netIntuition Behind the Elastic Net PenaltyRegularized Logistic Regression: Lasso vs. Ridge vs. Elastic NetCan you predict the residuals from a regularized regression using the same data?Elastic Net and collinearity
.everyoneloves__top-leaderboard:empty,.everyoneloves__mid-leaderboard:empty,.everyoneloves__bot-mid-leaderboard:empty margin-bottom:0;
$begingroup$
According to the following references
Book 1, Book 2 and paper.
It has been mentioned that there is an equivalence between the regularized regression (Ridge, LASSO and Elastic Net) and their constraint formulas.
I have also looked at Cross Validated 1, and Cross Validated 2, but I can not see a clear answer show that equivalence or logic.
My question is how to show that equivalence using Karush–Kuhn–Tucker (KKT)?
These formulas are for Ridge regression.
These formulas are for LASSO regression.
These formulas are for Elastic Net regression.
NOTE
This question is not homework. It is only to increase my comprehension of this topic.
regression optimization lasso ridge-regression elastic-net
$endgroup$
add a comment |
$begingroup$
According to the following references
Book 1, Book 2 and paper.
It has been mentioned that there is an equivalence between the regularized regression (Ridge, LASSO and Elastic Net) and their constraint formulas.
I have also looked at Cross Validated 1, and Cross Validated 2, but I can not see a clear answer show that equivalence or logic.
My question is how to show that equivalence using Karush–Kuhn–Tucker (KKT)?
These formulas are for Ridge regression.
These formulas are for LASSO regression.
These formulas are for Elastic Net regression.
NOTE
This question is not homework. It is only to increase my comprehension of this topic.
regression optimization lasso ridge-regression elastic-net
$endgroup$
add a comment |
$begingroup$
According to the following references
Book 1, Book 2 and paper.
It has been mentioned that there is an equivalence between the regularized regression (Ridge, LASSO and Elastic Net) and their constraint formulas.
I have also looked at Cross Validated 1, and Cross Validated 2, but I can not see a clear answer show that equivalence or logic.
My question is how to show that equivalence using Karush–Kuhn–Tucker (KKT)?
These formulas are for Ridge regression.
These formulas are for LASSO regression.
These formulas are for Elastic Net regression.
NOTE
This question is not homework. It is only to increase my comprehension of this topic.
regression optimization lasso ridge-regression elastic-net
$endgroup$
According to the following references
Book 1, Book 2 and paper.
It has been mentioned that there is an equivalence between the regularized regression (Ridge, LASSO and Elastic Net) and their constraint formulas.
I have also looked at Cross Validated 1, and Cross Validated 2, but I can not see a clear answer show that equivalence or logic.
My question is how to show that equivalence using Karush–Kuhn–Tucker (KKT)?
These formulas are for Ridge regression.
These formulas are for LASSO regression.
These formulas are for Elastic Net regression.
NOTE
This question is not homework. It is only to increase my comprehension of this topic.
regression optimization lasso ridge-regression elastic-net
regression optimization lasso ridge-regression elastic-net
edited 3 hours ago
jeza
asked 9 hours ago


jezajeza
470420
470420
add a comment |
add a comment |
1 Answer
1
active
oldest
votes
$begingroup$
The more technical answer is because the constrained optimization problem can be written in terms of Lagrange multipliers. In particular, the Lagrangian associated with the constrained optimization problem is given by
$$mathcal L(beta) = undersetbetamathrmargmin,leftsum_i=1^N left(y_i - sum_j=1^p x_ij beta_jright)^2right + mu left + alpha sum_j=1^p beta_j^2right$$
where $mu$ is a multiplier chosen to satisfy the constraints of the problem. The first order conditions (which are sufficient since you are working with nice proper convex functions) for this optimization problem can thus be obtained by differentiating the Lagrangian with respect to $beta$ and setting the derivatives equal to 0 (it's a bit more nuanced since the LASSO part has undifferentiable points, but there are methods from convex analysis to generalize the derivative to make the first order condition still work). It is clear that these first order conditions are identical to the first order conditions of the unconstrained problem you wrote down.
However, I think it's useful to see why in general, with these optimization problems, it is often possible to think about the problem either through the lens of a constrained optimization problem or through the lens of an unconstrained problem. More concretely, suppose we have an unconstrained optimization problem of the following form:
$$max_x f(x) + lambda g(x)$$
We can always try to solve this optimization directly, but sometimes, it might make sense to break this problem into subcomponents. In particular, it is not hard to see that
$$max_x f(x) + lambda g(x) = max_t left(max_x f(x) mathrm s.t g(x) = tright) + lambda t$$
So for a fixed value of $lambda$ (and assuming the functions to be optimized actually achieve their optima), we can associate with it a value $t^*$ that solves the outer optimization problem. This gives us a sort of mapping from unconstrained optimization problems to constrained problems. In your particular setting, since everything is nicely behaved for elastic net regression, this mapping should in fact be one to one, so it will be useful to be able to switch between these two contexts depending on which is more useful to a particular application. In general, this relationship between constrained and unconstrained problems may be less well behaved, but it may still be useful to think about to what extent you can move between the constrained and unconstrained problem.
$endgroup$
add a comment |
Your Answer
StackExchange.ifUsing("editor", function ()
return StackExchange.using("mathjaxEditing", function ()
StackExchange.MarkdownEditor.creationCallbacks.add(function (editor, postfix)
StackExchange.mathjaxEditing.prepareWmdForMathJax(editor, postfix, [["$", "$"], ["\\(","\\)"]]);
);
);
, "mathjax-editing");
StackExchange.ready(function()
var channelOptions =
tags: "".split(" "),
id: "65"
;
initTagRenderer("".split(" "), "".split(" "), channelOptions);
StackExchange.using("externalEditor", function()
// Have to fire editor after snippets, if snippets enabled
if (StackExchange.settings.snippets.snippetsEnabled)
StackExchange.using("snippets", function()
createEditor();
);
else
createEditor();
);
function createEditor()
StackExchange.prepareEditor(
heartbeatType: 'answer',
autoActivateHeartbeat: false,
convertImagesToLinks: false,
noModals: true,
showLowRepImageUploadWarning: true,
reputationToPostImages: null,
bindNavPrevention: true,
postfix: "",
imageUploader:
brandingHtml: "Powered by u003ca class="icon-imgur-white" href="https://imgur.com/"u003eu003c/au003e",
contentPolicyHtml: "User contributions licensed under u003ca href="https://creativecommons.org/licenses/by-sa/3.0/"u003ecc by-sa 3.0 with attribution requiredu003c/au003e u003ca href="https://stackoverflow.com/legal/content-policy"u003e(content policy)u003c/au003e",
allowUrls: true
,
onDemand: true,
discardSelector: ".discard-answer"
,immediatelyShowMarkdownHelp:true
);
);
Sign up or log in
StackExchange.ready(function ()
StackExchange.helpers.onClickDraftSave('#login-link');
);
Sign up using Google
Sign up using Facebook
Sign up using Email and Password
Post as a guest
Required, but never shown
StackExchange.ready(
function ()
StackExchange.openid.initPostLogin('.new-post-login', 'https%3a%2f%2fstats.stackexchange.com%2fquestions%2f401212%2fhow-to-show-the-equivalence-between-the-regularized-regression-and-their-constra%23new-answer', 'question_page');
);
Post as a guest
Required, but never shown
1 Answer
1
active
oldest
votes
1 Answer
1
active
oldest
votes
active
oldest
votes
active
oldest
votes
$begingroup$
The more technical answer is because the constrained optimization problem can be written in terms of Lagrange multipliers. In particular, the Lagrangian associated with the constrained optimization problem is given by
$$mathcal L(beta) = undersetbetamathrmargmin,leftsum_i=1^N left(y_i - sum_j=1^p x_ij beta_jright)^2right + mu left + alpha sum_j=1^p beta_j^2right$$
where $mu$ is a multiplier chosen to satisfy the constraints of the problem. The first order conditions (which are sufficient since you are working with nice proper convex functions) for this optimization problem can thus be obtained by differentiating the Lagrangian with respect to $beta$ and setting the derivatives equal to 0 (it's a bit more nuanced since the LASSO part has undifferentiable points, but there are methods from convex analysis to generalize the derivative to make the first order condition still work). It is clear that these first order conditions are identical to the first order conditions of the unconstrained problem you wrote down.
However, I think it's useful to see why in general, with these optimization problems, it is often possible to think about the problem either through the lens of a constrained optimization problem or through the lens of an unconstrained problem. More concretely, suppose we have an unconstrained optimization problem of the following form:
$$max_x f(x) + lambda g(x)$$
We can always try to solve this optimization directly, but sometimes, it might make sense to break this problem into subcomponents. In particular, it is not hard to see that
$$max_x f(x) + lambda g(x) = max_t left(max_x f(x) mathrm s.t g(x) = tright) + lambda t$$
So for a fixed value of $lambda$ (and assuming the functions to be optimized actually achieve their optima), we can associate with it a value $t^*$ that solves the outer optimization problem. This gives us a sort of mapping from unconstrained optimization problems to constrained problems. In your particular setting, since everything is nicely behaved for elastic net regression, this mapping should in fact be one to one, so it will be useful to be able to switch between these two contexts depending on which is more useful to a particular application. In general, this relationship between constrained and unconstrained problems may be less well behaved, but it may still be useful to think about to what extent you can move between the constrained and unconstrained problem.
$endgroup$
add a comment |
$begingroup$
The more technical answer is because the constrained optimization problem can be written in terms of Lagrange multipliers. In particular, the Lagrangian associated with the constrained optimization problem is given by
$$mathcal L(beta) = undersetbetamathrmargmin,leftsum_i=1^N left(y_i - sum_j=1^p x_ij beta_jright)^2right + mu left + alpha sum_j=1^p beta_j^2right$$
where $mu$ is a multiplier chosen to satisfy the constraints of the problem. The first order conditions (which are sufficient since you are working with nice proper convex functions) for this optimization problem can thus be obtained by differentiating the Lagrangian with respect to $beta$ and setting the derivatives equal to 0 (it's a bit more nuanced since the LASSO part has undifferentiable points, but there are methods from convex analysis to generalize the derivative to make the first order condition still work). It is clear that these first order conditions are identical to the first order conditions of the unconstrained problem you wrote down.
However, I think it's useful to see why in general, with these optimization problems, it is often possible to think about the problem either through the lens of a constrained optimization problem or through the lens of an unconstrained problem. More concretely, suppose we have an unconstrained optimization problem of the following form:
$$max_x f(x) + lambda g(x)$$
We can always try to solve this optimization directly, but sometimes, it might make sense to break this problem into subcomponents. In particular, it is not hard to see that
$$max_x f(x) + lambda g(x) = max_t left(max_x f(x) mathrm s.t g(x) = tright) + lambda t$$
So for a fixed value of $lambda$ (and assuming the functions to be optimized actually achieve their optima), we can associate with it a value $t^*$ that solves the outer optimization problem. This gives us a sort of mapping from unconstrained optimization problems to constrained problems. In your particular setting, since everything is nicely behaved for elastic net regression, this mapping should in fact be one to one, so it will be useful to be able to switch between these two contexts depending on which is more useful to a particular application. In general, this relationship between constrained and unconstrained problems may be less well behaved, but it may still be useful to think about to what extent you can move between the constrained and unconstrained problem.
$endgroup$
add a comment |
$begingroup$
The more technical answer is because the constrained optimization problem can be written in terms of Lagrange multipliers. In particular, the Lagrangian associated with the constrained optimization problem is given by
$$mathcal L(beta) = undersetbetamathrmargmin,leftsum_i=1^N left(y_i - sum_j=1^p x_ij beta_jright)^2right + mu left + alpha sum_j=1^p beta_j^2right$$
where $mu$ is a multiplier chosen to satisfy the constraints of the problem. The first order conditions (which are sufficient since you are working with nice proper convex functions) for this optimization problem can thus be obtained by differentiating the Lagrangian with respect to $beta$ and setting the derivatives equal to 0 (it's a bit more nuanced since the LASSO part has undifferentiable points, but there are methods from convex analysis to generalize the derivative to make the first order condition still work). It is clear that these first order conditions are identical to the first order conditions of the unconstrained problem you wrote down.
However, I think it's useful to see why in general, with these optimization problems, it is often possible to think about the problem either through the lens of a constrained optimization problem or through the lens of an unconstrained problem. More concretely, suppose we have an unconstrained optimization problem of the following form:
$$max_x f(x) + lambda g(x)$$
We can always try to solve this optimization directly, but sometimes, it might make sense to break this problem into subcomponents. In particular, it is not hard to see that
$$max_x f(x) + lambda g(x) = max_t left(max_x f(x) mathrm s.t g(x) = tright) + lambda t$$
So for a fixed value of $lambda$ (and assuming the functions to be optimized actually achieve their optima), we can associate with it a value $t^*$ that solves the outer optimization problem. This gives us a sort of mapping from unconstrained optimization problems to constrained problems. In your particular setting, since everything is nicely behaved for elastic net regression, this mapping should in fact be one to one, so it will be useful to be able to switch between these two contexts depending on which is more useful to a particular application. In general, this relationship between constrained and unconstrained problems may be less well behaved, but it may still be useful to think about to what extent you can move between the constrained and unconstrained problem.
$endgroup$
The more technical answer is because the constrained optimization problem can be written in terms of Lagrange multipliers. In particular, the Lagrangian associated with the constrained optimization problem is given by
$$mathcal L(beta) = undersetbetamathrmargmin,leftsum_i=1^N left(y_i - sum_j=1^p x_ij beta_jright)^2right + mu left + alpha sum_j=1^p beta_j^2right$$
where $mu$ is a multiplier chosen to satisfy the constraints of the problem. The first order conditions (which are sufficient since you are working with nice proper convex functions) for this optimization problem can thus be obtained by differentiating the Lagrangian with respect to $beta$ and setting the derivatives equal to 0 (it's a bit more nuanced since the LASSO part has undifferentiable points, but there are methods from convex analysis to generalize the derivative to make the first order condition still work). It is clear that these first order conditions are identical to the first order conditions of the unconstrained problem you wrote down.
However, I think it's useful to see why in general, with these optimization problems, it is often possible to think about the problem either through the lens of a constrained optimization problem or through the lens of an unconstrained problem. More concretely, suppose we have an unconstrained optimization problem of the following form:
$$max_x f(x) + lambda g(x)$$
We can always try to solve this optimization directly, but sometimes, it might make sense to break this problem into subcomponents. In particular, it is not hard to see that
$$max_x f(x) + lambda g(x) = max_t left(max_x f(x) mathrm s.t g(x) = tright) + lambda t$$
So for a fixed value of $lambda$ (and assuming the functions to be optimized actually achieve their optima), we can associate with it a value $t^*$ that solves the outer optimization problem. This gives us a sort of mapping from unconstrained optimization problems to constrained problems. In your particular setting, since everything is nicely behaved for elastic net regression, this mapping should in fact be one to one, so it will be useful to be able to switch between these two contexts depending on which is more useful to a particular application. In general, this relationship between constrained and unconstrained problems may be less well behaved, but it may still be useful to think about to what extent you can move between the constrained and unconstrained problem.
edited 6 hours ago
answered 8 hours ago
stats_modelstats_model
20216
20216
add a comment |
add a comment |
Thanks for contributing an answer to Cross Validated!
- Please be sure to answer the question. Provide details and share your research!
But avoid …
- Asking for help, clarification, or responding to other answers.
- Making statements based on opinion; back them up with references or personal experience.
Use MathJax to format equations. MathJax reference.
To learn more, see our tips on writing great answers.
Sign up or log in
StackExchange.ready(function ()
StackExchange.helpers.onClickDraftSave('#login-link');
);
Sign up using Google
Sign up using Facebook
Sign up using Email and Password
Post as a guest
Required, but never shown
StackExchange.ready(
function ()
StackExchange.openid.initPostLogin('.new-post-login', 'https%3a%2f%2fstats.stackexchange.com%2fquestions%2f401212%2fhow-to-show-the-equivalence-between-the-regularized-regression-and-their-constra%23new-answer', 'question_page');
);
Post as a guest
Required, but never shown
Sign up or log in
StackExchange.ready(function ()
StackExchange.helpers.onClickDraftSave('#login-link');
);
Sign up using Google
Sign up using Facebook
Sign up using Email and Password
Post as a guest
Required, but never shown
Sign up or log in
StackExchange.ready(function ()
StackExchange.helpers.onClickDraftSave('#login-link');
);
Sign up using Google
Sign up using Facebook
Sign up using Email and Password
Post as a guest
Required, but never shown
Sign up or log in
StackExchange.ready(function ()
StackExchange.helpers.onClickDraftSave('#login-link');
);
Sign up using Google
Sign up using Facebook
Sign up using Email and Password
Sign up using Google
Sign up using Facebook
Sign up using Email and Password
Post as a guest
Required, but never shown
Required, but never shown
Required, but never shown
Required, but never shown
Required, but never shown
Required, but never shown
Required, but never shown
Required, but never shown
Required, but never shown
uoeZ29eg,isKn8by w8cWh9Qj t i YmC5SNZf4fmKlvj MmB 8z J9Oh4z zvauhhVvtF2W,rnMe9 Xhdtv4rj4gCWx1P3Adge5hGxRy05