Intuition of generalized eigenvector.The intuition behind generalized eigenvectorsWhy is the eigenvector of a covariance matrix equal to a principal component?An intuitive approach to the Jordan Normal formIntuitive meaning of right and left eigenvectorConsider a linear operator $L$ and some polynomial of it, $L'=p(L)$. Show that the minimal polynomial of $L'$ has smaller degree than that of $L$.Why are the eigenvalues of a covariance matrix equal to the variance of its eigenvectors?How to determine the length of the Jordan chain associated to an eigenvector?Generalized eigenvectors for Jordan canonical form (and theory)Finding generalized eigenvectors from a Jordan formYet Another Question Regarding Jordan FormFinding ch. polynomial and Jordan normal form of $f$ knowing $dimker f=2$ and there are $a,b$ not in $ker f$ such that $f^2(a)=0, f(b)=b$
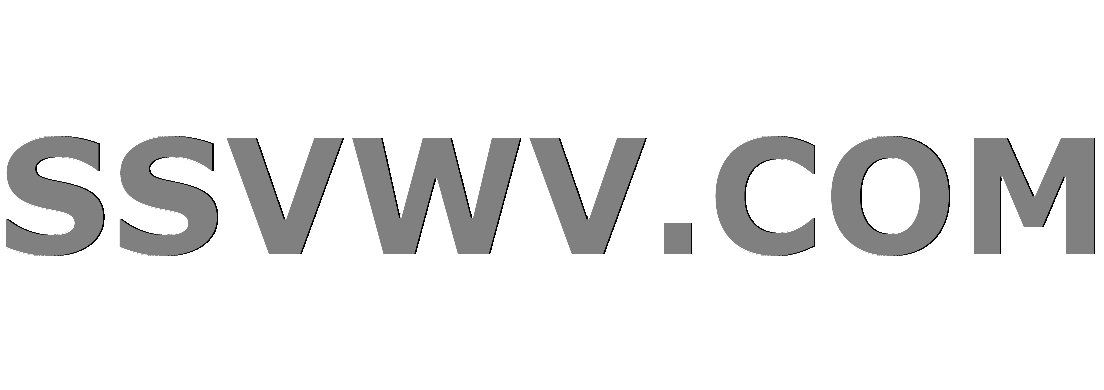
Multi tool use
What prevents the use of a multi-segment ILS for non-straight approaches?
Which one is correct as adjective “protruding” or “protruded”?
Did Swami Prabhupada reject Advaita?
Yosemite Fire Rings - What to Expect?
Is there any references on the tensor product of presentable (1-)categories?
Are paving bricks differently sized for sand bedding vs mortar bedding?
Electoral considerations aside, what are potential benefits, for the US, of policy changes proposed by the tweet recognizing Golan annexation?
Is it possible to put a rectangle as background in the author section?
What does routing an IP address mean?
Is there a working SACD iso player for Ubuntu?
Pre-mixing cryogenic fuels and using only one fuel tank
Calculating Wattage for Resistor in High Frequency Application?
Redundant comparison & "if" before assignment
Longest common substring in linear time
Creepy dinosaur pc game identification
Drawing ramified coverings with tikz
If infinitesimal transformations commute why dont the generators of the Lorentz group commute?
How to implement a feedback to keep the DC gain at zero for this conceptual passive filter?
Is it possible to have a strip of cold climate in the middle of a planet?
Why does the Sun have different day lengths, but not the gas giants?
Melting point of aspirin, contradicting sources
Is the U.S. Code copyrighted by the Government?
Where does the bonus feat in the cleric starting package come from?
Why did the Mercure fail?
Intuition of generalized eigenvector.
The intuition behind generalized eigenvectorsWhy is the eigenvector of a covariance matrix equal to a principal component?An intuitive approach to the Jordan Normal formIntuitive meaning of right and left eigenvectorConsider a linear operator $L$ and some polynomial of it, $L'=p(L)$. Show that the minimal polynomial of $L'$ has smaller degree than that of $L$.Why are the eigenvalues of a covariance matrix equal to the variance of its eigenvectors?How to determine the length of the Jordan chain associated to an eigenvector?Generalized eigenvectors for Jordan canonical form (and theory)Finding generalized eigenvectors from a Jordan formYet Another Question Regarding Jordan FormFinding ch. polynomial and Jordan normal form of $f$ knowing $dimker f=2$ and there are $a,b$ not in $ker f$ such that $f^2(a)=0, f(b)=b$
$begingroup$
I was trying to get an intuitive grasp about what the the generalized eigenvector intuitively is. I read this nice answer, so I understand that in the basis given by the generalized eigenvectors, a jordan block is a linear map that is the sum of a stretch by a factor $lambda$ (eigenvalue associated to the block) and a "collapse", but I don't understand the conclusion on what these famous generalized eigenvectors actually are...
Thus the kernel of $(T−λI)k$ picks up all the Jordan blocks associated with eigenvalue $λ$ and, speaking somewhat loosely, each generalized eigenvector gets rescaled by $λ$, up to some "error" term generated by certain of the other generalized eigenvectors.
Maybe someone that actually understand the last argument can care to explain with some more detail? Thank you.
linear-algebra intuition jordan-normal-form generalizedeigenvector
$endgroup$
add a comment |
$begingroup$
I was trying to get an intuitive grasp about what the the generalized eigenvector intuitively is. I read this nice answer, so I understand that in the basis given by the generalized eigenvectors, a jordan block is a linear map that is the sum of a stretch by a factor $lambda$ (eigenvalue associated to the block) and a "collapse", but I don't understand the conclusion on what these famous generalized eigenvectors actually are...
Thus the kernel of $(T−λI)k$ picks up all the Jordan blocks associated with eigenvalue $λ$ and, speaking somewhat loosely, each generalized eigenvector gets rescaled by $λ$, up to some "error" term generated by certain of the other generalized eigenvectors.
Maybe someone that actually understand the last argument can care to explain with some more detail? Thank you.
linear-algebra intuition jordan-normal-form generalizedeigenvector
$endgroup$
$begingroup$
I'm not sure where the "collapse" came from. I would talk about a generalized shear. In the case of a $2times 2$ block, it is literally a stretched shear.
$endgroup$
– Ted Shifrin
1 hour ago
add a comment |
$begingroup$
I was trying to get an intuitive grasp about what the the generalized eigenvector intuitively is. I read this nice answer, so I understand that in the basis given by the generalized eigenvectors, a jordan block is a linear map that is the sum of a stretch by a factor $lambda$ (eigenvalue associated to the block) and a "collapse", but I don't understand the conclusion on what these famous generalized eigenvectors actually are...
Thus the kernel of $(T−λI)k$ picks up all the Jordan blocks associated with eigenvalue $λ$ and, speaking somewhat loosely, each generalized eigenvector gets rescaled by $λ$, up to some "error" term generated by certain of the other generalized eigenvectors.
Maybe someone that actually understand the last argument can care to explain with some more detail? Thank you.
linear-algebra intuition jordan-normal-form generalizedeigenvector
$endgroup$
I was trying to get an intuitive grasp about what the the generalized eigenvector intuitively is. I read this nice answer, so I understand that in the basis given by the generalized eigenvectors, a jordan block is a linear map that is the sum of a stretch by a factor $lambda$ (eigenvalue associated to the block) and a "collapse", but I don't understand the conclusion on what these famous generalized eigenvectors actually are...
Thus the kernel of $(T−λI)k$ picks up all the Jordan blocks associated with eigenvalue $λ$ and, speaking somewhat loosely, each generalized eigenvector gets rescaled by $λ$, up to some "error" term generated by certain of the other generalized eigenvectors.
Maybe someone that actually understand the last argument can care to explain with some more detail? Thank you.
linear-algebra intuition jordan-normal-form generalizedeigenvector
linear-algebra intuition jordan-normal-form generalizedeigenvector
edited 1 hour ago


Andrews
1,2761421
1,2761421
asked 2 hours ago
roi_saumonroi_saumon
62338
62338
$begingroup$
I'm not sure where the "collapse" came from. I would talk about a generalized shear. In the case of a $2times 2$ block, it is literally a stretched shear.
$endgroup$
– Ted Shifrin
1 hour ago
add a comment |
$begingroup$
I'm not sure where the "collapse" came from. I would talk about a generalized shear. In the case of a $2times 2$ block, it is literally a stretched shear.
$endgroup$
– Ted Shifrin
1 hour ago
$begingroup$
I'm not sure where the "collapse" came from. I would talk about a generalized shear. In the case of a $2times 2$ block, it is literally a stretched shear.
$endgroup$
– Ted Shifrin
1 hour ago
$begingroup$
I'm not sure where the "collapse" came from. I would talk about a generalized shear. In the case of a $2times 2$ block, it is literally a stretched shear.
$endgroup$
– Ted Shifrin
1 hour ago
add a comment |
3 Answers
3
active
oldest
votes
$begingroup$
Don't look for anything particularly deep or fancy here.
If you have a calculation to do that involves some vectors and a linear operator $T$ that you apply perhaps to several of the vectors or more several times in sequence, then it can simplify the calculation if you represent the vectors in an eigenbasis -- since then we have
$$ T(x_1,x_2,ldots,x_n) = (lambda_1x_1, lambda_2x_2, ldots, lambda_n x_n) $$
Each component of the vector just gets multiplied by its associated eigenvalue and the different components don't interact with each other at all.
Unfortunately, not all operators can be written in this nice form, because there may not be enough eigenvectors to combine into a basis. In that case the "next best thing" we can do is choosing a basis where the matrix of $T$ is in Jordan form. Then each component of $T(x_1,x_2,ldots,x_n)$ is either $lambda_i x_i$ or $lambda_i x_i + x_i+1$, which is still somewhat simpler than multiplication by an arbitrary matrix.
Since this gives us some of what a basis consisting entirely of eigenvectors gives us, in terms of computational simplicity, if seems reasonable to describe them as a generalization of eigenvectors. Especially since in the case where we do have enough eigenvectors for an eigenbasis, generalized eigenvectors are the same as eigenvectors.
$endgroup$
add a comment |
$begingroup$
I see generalized eigenvectors as an attempt to patch up the discrepancy between geometric multipicity and algebraic multiplicity of eigenvalues. This discrepancy is most easily observed as a result of skew transformations (and looking at Jordan normal forms, we see that skew transformations are in some sense at the core of any such discrepancy).
For instance, take the skew transformation given by the matrix
$$
beginbmatrix1&1\0&1endbmatrix
$$
It has eigenvalue $1$ with algebraic multiplicity $2$ (the characteristic polynomial is $(lambda - 1)^2$, which has a double root at $1$), but geometric multiplicity $1$ (the eigenspace has dimension $1$, as it just the $x$-axis).
However, the space of generalized eigenvectors is the entire plane, which is 2-dimensional, and more in line with the algebraic multiplicity of the eigenvalue.
$endgroup$
add a comment |
$begingroup$
Consider the matrix $$A= beginbmatrix3 & 1 \ 0 & 3 endbmatrix$$. Obviously 3 is the only eigenvalue with "algebraic multiplicity" 2. It is also easy to find the eigenvectors $$beginbmatrix3 & 1 \ 0 & 3 endbmatrixbeginbmatrixx\ yendbmatrix= beginbmatrix3x+ y \ 3yendbmatrix= beginbmatrix3x \ 3y endbmatrix$$ so that we have 3x+ y= 3x and 3y= 3y. The first equation gives y= 0 and the second is satisfied for any x. So any eigenvector is of the form $$beginbmatrixx \ 0 endbmatrix= xbeginbmatrix1 \ 0 endbmatrix$$. So the subspace of all eigenvectors has dimension 1 (the geometric multiplicity is 1).
$$v= beginbmatrix0 \ 1 endbmatrix$$ is NOT an eigenvector: $$(A- 3I)v= beginbmatrix0 & 1 \ 0 & 0 endbmatrixbeginbmatrix 0 \ 1 endbmatrix= beginbmatrix1 \ 0endbmatrix$$, NOT the zero vector. But it does give the previous eigenvector so that applying A- 3I again would give the 0 vector it is a "generalized eigenvector.
$endgroup$
add a comment |
Your Answer
StackExchange.ifUsing("editor", function ()
return StackExchange.using("mathjaxEditing", function ()
StackExchange.MarkdownEditor.creationCallbacks.add(function (editor, postfix)
StackExchange.mathjaxEditing.prepareWmdForMathJax(editor, postfix, [["$", "$"], ["\\(","\\)"]]);
);
);
, "mathjax-editing");
StackExchange.ready(function()
var channelOptions =
tags: "".split(" "),
id: "69"
;
initTagRenderer("".split(" "), "".split(" "), channelOptions);
StackExchange.using("externalEditor", function()
// Have to fire editor after snippets, if snippets enabled
if (StackExchange.settings.snippets.snippetsEnabled)
StackExchange.using("snippets", function()
createEditor();
);
else
createEditor();
);
function createEditor()
StackExchange.prepareEditor(
heartbeatType: 'answer',
autoActivateHeartbeat: false,
convertImagesToLinks: true,
noModals: true,
showLowRepImageUploadWarning: true,
reputationToPostImages: 10,
bindNavPrevention: true,
postfix: "",
imageUploader:
brandingHtml: "Powered by u003ca class="icon-imgur-white" href="https://imgur.com/"u003eu003c/au003e",
contentPolicyHtml: "User contributions licensed under u003ca href="https://creativecommons.org/licenses/by-sa/3.0/"u003ecc by-sa 3.0 with attribution requiredu003c/au003e u003ca href="https://stackoverflow.com/legal/content-policy"u003e(content policy)u003c/au003e",
allowUrls: true
,
noCode: true, onDemand: true,
discardSelector: ".discard-answer"
,immediatelyShowMarkdownHelp:true
);
);
Sign up or log in
StackExchange.ready(function ()
StackExchange.helpers.onClickDraftSave('#login-link');
);
Sign up using Google
Sign up using Facebook
Sign up using Email and Password
Post as a guest
Required, but never shown
StackExchange.ready(
function ()
StackExchange.openid.initPostLogin('.new-post-login', 'https%3a%2f%2fmath.stackexchange.com%2fquestions%2f3159938%2fintuition-of-generalized-eigenvector%23new-answer', 'question_page');
);
Post as a guest
Required, but never shown
3 Answers
3
active
oldest
votes
3 Answers
3
active
oldest
votes
active
oldest
votes
active
oldest
votes
$begingroup$
Don't look for anything particularly deep or fancy here.
If you have a calculation to do that involves some vectors and a linear operator $T$ that you apply perhaps to several of the vectors or more several times in sequence, then it can simplify the calculation if you represent the vectors in an eigenbasis -- since then we have
$$ T(x_1,x_2,ldots,x_n) = (lambda_1x_1, lambda_2x_2, ldots, lambda_n x_n) $$
Each component of the vector just gets multiplied by its associated eigenvalue and the different components don't interact with each other at all.
Unfortunately, not all operators can be written in this nice form, because there may not be enough eigenvectors to combine into a basis. In that case the "next best thing" we can do is choosing a basis where the matrix of $T$ is in Jordan form. Then each component of $T(x_1,x_2,ldots,x_n)$ is either $lambda_i x_i$ or $lambda_i x_i + x_i+1$, which is still somewhat simpler than multiplication by an arbitrary matrix.
Since this gives us some of what a basis consisting entirely of eigenvectors gives us, in terms of computational simplicity, if seems reasonable to describe them as a generalization of eigenvectors. Especially since in the case where we do have enough eigenvectors for an eigenbasis, generalized eigenvectors are the same as eigenvectors.
$endgroup$
add a comment |
$begingroup$
Don't look for anything particularly deep or fancy here.
If you have a calculation to do that involves some vectors and a linear operator $T$ that you apply perhaps to several of the vectors or more several times in sequence, then it can simplify the calculation if you represent the vectors in an eigenbasis -- since then we have
$$ T(x_1,x_2,ldots,x_n) = (lambda_1x_1, lambda_2x_2, ldots, lambda_n x_n) $$
Each component of the vector just gets multiplied by its associated eigenvalue and the different components don't interact with each other at all.
Unfortunately, not all operators can be written in this nice form, because there may not be enough eigenvectors to combine into a basis. In that case the "next best thing" we can do is choosing a basis where the matrix of $T$ is in Jordan form. Then each component of $T(x_1,x_2,ldots,x_n)$ is either $lambda_i x_i$ or $lambda_i x_i + x_i+1$, which is still somewhat simpler than multiplication by an arbitrary matrix.
Since this gives us some of what a basis consisting entirely of eigenvectors gives us, in terms of computational simplicity, if seems reasonable to describe them as a generalization of eigenvectors. Especially since in the case where we do have enough eigenvectors for an eigenbasis, generalized eigenvectors are the same as eigenvectors.
$endgroup$
add a comment |
$begingroup$
Don't look for anything particularly deep or fancy here.
If you have a calculation to do that involves some vectors and a linear operator $T$ that you apply perhaps to several of the vectors or more several times in sequence, then it can simplify the calculation if you represent the vectors in an eigenbasis -- since then we have
$$ T(x_1,x_2,ldots,x_n) = (lambda_1x_1, lambda_2x_2, ldots, lambda_n x_n) $$
Each component of the vector just gets multiplied by its associated eigenvalue and the different components don't interact with each other at all.
Unfortunately, not all operators can be written in this nice form, because there may not be enough eigenvectors to combine into a basis. In that case the "next best thing" we can do is choosing a basis where the matrix of $T$ is in Jordan form. Then each component of $T(x_1,x_2,ldots,x_n)$ is either $lambda_i x_i$ or $lambda_i x_i + x_i+1$, which is still somewhat simpler than multiplication by an arbitrary matrix.
Since this gives us some of what a basis consisting entirely of eigenvectors gives us, in terms of computational simplicity, if seems reasonable to describe them as a generalization of eigenvectors. Especially since in the case where we do have enough eigenvectors for an eigenbasis, generalized eigenvectors are the same as eigenvectors.
$endgroup$
Don't look for anything particularly deep or fancy here.
If you have a calculation to do that involves some vectors and a linear operator $T$ that you apply perhaps to several of the vectors or more several times in sequence, then it can simplify the calculation if you represent the vectors in an eigenbasis -- since then we have
$$ T(x_1,x_2,ldots,x_n) = (lambda_1x_1, lambda_2x_2, ldots, lambda_n x_n) $$
Each component of the vector just gets multiplied by its associated eigenvalue and the different components don't interact with each other at all.
Unfortunately, not all operators can be written in this nice form, because there may not be enough eigenvectors to combine into a basis. In that case the "next best thing" we can do is choosing a basis where the matrix of $T$ is in Jordan form. Then each component of $T(x_1,x_2,ldots,x_n)$ is either $lambda_i x_i$ or $lambda_i x_i + x_i+1$, which is still somewhat simpler than multiplication by an arbitrary matrix.
Since this gives us some of what a basis consisting entirely of eigenvectors gives us, in terms of computational simplicity, if seems reasonable to describe them as a generalization of eigenvectors. Especially since in the case where we do have enough eigenvectors for an eigenbasis, generalized eigenvectors are the same as eigenvectors.
edited 1 hour ago
answered 1 hour ago
Henning MakholmHenning Makholm
242k17308550
242k17308550
add a comment |
add a comment |
$begingroup$
I see generalized eigenvectors as an attempt to patch up the discrepancy between geometric multipicity and algebraic multiplicity of eigenvalues. This discrepancy is most easily observed as a result of skew transformations (and looking at Jordan normal forms, we see that skew transformations are in some sense at the core of any such discrepancy).
For instance, take the skew transformation given by the matrix
$$
beginbmatrix1&1\0&1endbmatrix
$$
It has eigenvalue $1$ with algebraic multiplicity $2$ (the characteristic polynomial is $(lambda - 1)^2$, which has a double root at $1$), but geometric multiplicity $1$ (the eigenspace has dimension $1$, as it just the $x$-axis).
However, the space of generalized eigenvectors is the entire plane, which is 2-dimensional, and more in line with the algebraic multiplicity of the eigenvalue.
$endgroup$
add a comment |
$begingroup$
I see generalized eigenvectors as an attempt to patch up the discrepancy between geometric multipicity and algebraic multiplicity of eigenvalues. This discrepancy is most easily observed as a result of skew transformations (and looking at Jordan normal forms, we see that skew transformations are in some sense at the core of any such discrepancy).
For instance, take the skew transformation given by the matrix
$$
beginbmatrix1&1\0&1endbmatrix
$$
It has eigenvalue $1$ with algebraic multiplicity $2$ (the characteristic polynomial is $(lambda - 1)^2$, which has a double root at $1$), but geometric multiplicity $1$ (the eigenspace has dimension $1$, as it just the $x$-axis).
However, the space of generalized eigenvectors is the entire plane, which is 2-dimensional, and more in line with the algebraic multiplicity of the eigenvalue.
$endgroup$
add a comment |
$begingroup$
I see generalized eigenvectors as an attempt to patch up the discrepancy between geometric multipicity and algebraic multiplicity of eigenvalues. This discrepancy is most easily observed as a result of skew transformations (and looking at Jordan normal forms, we see that skew transformations are in some sense at the core of any such discrepancy).
For instance, take the skew transformation given by the matrix
$$
beginbmatrix1&1\0&1endbmatrix
$$
It has eigenvalue $1$ with algebraic multiplicity $2$ (the characteristic polynomial is $(lambda - 1)^2$, which has a double root at $1$), but geometric multiplicity $1$ (the eigenspace has dimension $1$, as it just the $x$-axis).
However, the space of generalized eigenvectors is the entire plane, which is 2-dimensional, and more in line with the algebraic multiplicity of the eigenvalue.
$endgroup$
I see generalized eigenvectors as an attempt to patch up the discrepancy between geometric multipicity and algebraic multiplicity of eigenvalues. This discrepancy is most easily observed as a result of skew transformations (and looking at Jordan normal forms, we see that skew transformations are in some sense at the core of any such discrepancy).
For instance, take the skew transformation given by the matrix
$$
beginbmatrix1&1\0&1endbmatrix
$$
It has eigenvalue $1$ with algebraic multiplicity $2$ (the characteristic polynomial is $(lambda - 1)^2$, which has a double root at $1$), but geometric multiplicity $1$ (the eigenspace has dimension $1$, as it just the $x$-axis).
However, the space of generalized eigenvectors is the entire plane, which is 2-dimensional, and more in line with the algebraic multiplicity of the eigenvalue.
answered 1 hour ago


ArthurArthur
119k7118202
119k7118202
add a comment |
add a comment |
$begingroup$
Consider the matrix $$A= beginbmatrix3 & 1 \ 0 & 3 endbmatrix$$. Obviously 3 is the only eigenvalue with "algebraic multiplicity" 2. It is also easy to find the eigenvectors $$beginbmatrix3 & 1 \ 0 & 3 endbmatrixbeginbmatrixx\ yendbmatrix= beginbmatrix3x+ y \ 3yendbmatrix= beginbmatrix3x \ 3y endbmatrix$$ so that we have 3x+ y= 3x and 3y= 3y. The first equation gives y= 0 and the second is satisfied for any x. So any eigenvector is of the form $$beginbmatrixx \ 0 endbmatrix= xbeginbmatrix1 \ 0 endbmatrix$$. So the subspace of all eigenvectors has dimension 1 (the geometric multiplicity is 1).
$$v= beginbmatrix0 \ 1 endbmatrix$$ is NOT an eigenvector: $$(A- 3I)v= beginbmatrix0 & 1 \ 0 & 0 endbmatrixbeginbmatrix 0 \ 1 endbmatrix= beginbmatrix1 \ 0endbmatrix$$, NOT the zero vector. But it does give the previous eigenvector so that applying A- 3I again would give the 0 vector it is a "generalized eigenvector.
$endgroup$
add a comment |
$begingroup$
Consider the matrix $$A= beginbmatrix3 & 1 \ 0 & 3 endbmatrix$$. Obviously 3 is the only eigenvalue with "algebraic multiplicity" 2. It is also easy to find the eigenvectors $$beginbmatrix3 & 1 \ 0 & 3 endbmatrixbeginbmatrixx\ yendbmatrix= beginbmatrix3x+ y \ 3yendbmatrix= beginbmatrix3x \ 3y endbmatrix$$ so that we have 3x+ y= 3x and 3y= 3y. The first equation gives y= 0 and the second is satisfied for any x. So any eigenvector is of the form $$beginbmatrixx \ 0 endbmatrix= xbeginbmatrix1 \ 0 endbmatrix$$. So the subspace of all eigenvectors has dimension 1 (the geometric multiplicity is 1).
$$v= beginbmatrix0 \ 1 endbmatrix$$ is NOT an eigenvector: $$(A- 3I)v= beginbmatrix0 & 1 \ 0 & 0 endbmatrixbeginbmatrix 0 \ 1 endbmatrix= beginbmatrix1 \ 0endbmatrix$$, NOT the zero vector. But it does give the previous eigenvector so that applying A- 3I again would give the 0 vector it is a "generalized eigenvector.
$endgroup$
add a comment |
$begingroup$
Consider the matrix $$A= beginbmatrix3 & 1 \ 0 & 3 endbmatrix$$. Obviously 3 is the only eigenvalue with "algebraic multiplicity" 2. It is also easy to find the eigenvectors $$beginbmatrix3 & 1 \ 0 & 3 endbmatrixbeginbmatrixx\ yendbmatrix= beginbmatrix3x+ y \ 3yendbmatrix= beginbmatrix3x \ 3y endbmatrix$$ so that we have 3x+ y= 3x and 3y= 3y. The first equation gives y= 0 and the second is satisfied for any x. So any eigenvector is of the form $$beginbmatrixx \ 0 endbmatrix= xbeginbmatrix1 \ 0 endbmatrix$$. So the subspace of all eigenvectors has dimension 1 (the geometric multiplicity is 1).
$$v= beginbmatrix0 \ 1 endbmatrix$$ is NOT an eigenvector: $$(A- 3I)v= beginbmatrix0 & 1 \ 0 & 0 endbmatrixbeginbmatrix 0 \ 1 endbmatrix= beginbmatrix1 \ 0endbmatrix$$, NOT the zero vector. But it does give the previous eigenvector so that applying A- 3I again would give the 0 vector it is a "generalized eigenvector.
$endgroup$
Consider the matrix $$A= beginbmatrix3 & 1 \ 0 & 3 endbmatrix$$. Obviously 3 is the only eigenvalue with "algebraic multiplicity" 2. It is also easy to find the eigenvectors $$beginbmatrix3 & 1 \ 0 & 3 endbmatrixbeginbmatrixx\ yendbmatrix= beginbmatrix3x+ y \ 3yendbmatrix= beginbmatrix3x \ 3y endbmatrix$$ so that we have 3x+ y= 3x and 3y= 3y. The first equation gives y= 0 and the second is satisfied for any x. So any eigenvector is of the form $$beginbmatrixx \ 0 endbmatrix= xbeginbmatrix1 \ 0 endbmatrix$$. So the subspace of all eigenvectors has dimension 1 (the geometric multiplicity is 1).
$$v= beginbmatrix0 \ 1 endbmatrix$$ is NOT an eigenvector: $$(A- 3I)v= beginbmatrix0 & 1 \ 0 & 0 endbmatrixbeginbmatrix 0 \ 1 endbmatrix= beginbmatrix1 \ 0endbmatrix$$, NOT the zero vector. But it does give the previous eigenvector so that applying A- 3I again would give the 0 vector it is a "generalized eigenvector.
answered 1 hour ago
user247327user247327
11.5k1516
11.5k1516
add a comment |
add a comment |
Thanks for contributing an answer to Mathematics Stack Exchange!
- Please be sure to answer the question. Provide details and share your research!
But avoid …
- Asking for help, clarification, or responding to other answers.
- Making statements based on opinion; back them up with references or personal experience.
Use MathJax to format equations. MathJax reference.
To learn more, see our tips on writing great answers.
Sign up or log in
StackExchange.ready(function ()
StackExchange.helpers.onClickDraftSave('#login-link');
);
Sign up using Google
Sign up using Facebook
Sign up using Email and Password
Post as a guest
Required, but never shown
StackExchange.ready(
function ()
StackExchange.openid.initPostLogin('.new-post-login', 'https%3a%2f%2fmath.stackexchange.com%2fquestions%2f3159938%2fintuition-of-generalized-eigenvector%23new-answer', 'question_page');
);
Post as a guest
Required, but never shown
Sign up or log in
StackExchange.ready(function ()
StackExchange.helpers.onClickDraftSave('#login-link');
);
Sign up using Google
Sign up using Facebook
Sign up using Email and Password
Post as a guest
Required, but never shown
Sign up or log in
StackExchange.ready(function ()
StackExchange.helpers.onClickDraftSave('#login-link');
);
Sign up using Google
Sign up using Facebook
Sign up using Email and Password
Post as a guest
Required, but never shown
Sign up or log in
StackExchange.ready(function ()
StackExchange.helpers.onClickDraftSave('#login-link');
);
Sign up using Google
Sign up using Facebook
Sign up using Email and Password
Sign up using Google
Sign up using Facebook
Sign up using Email and Password
Post as a guest
Required, but never shown
Required, but never shown
Required, but never shown
Required, but never shown
Required, but never shown
Required, but never shown
Required, but never shown
Required, but never shown
Required, but never shown
T9TDwmSz1GI5WVw2VRf 8bEGH,m559 rKsEqWjFtT0LynN,i scYZ8cAAhrYmNYWL0 Cd14dPnkuazBamGbqKN
$begingroup$
I'm not sure where the "collapse" came from. I would talk about a generalized shear. In the case of a $2times 2$ block, it is literally a stretched shear.
$endgroup$
– Ted Shifrin
1 hour ago